Everything You Need to Know About AI Agents: What is AI Agent? (Part 1)
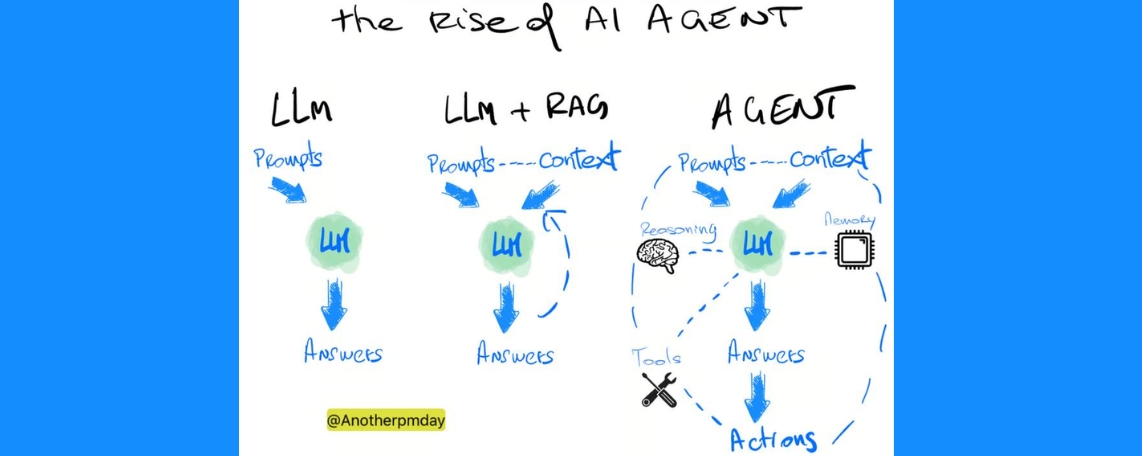
AI Agents: A New but Familiar Concept
While AI Agents may seem new, they are evolving existing technologies. In Vietnam, discussions around AI Agents started gaining traction at the end of 2024, but global reports from McKinsey, Gartner, Forbes, and Harvard Business Review have covered this trend since 2023.
To better understand AI Agents, let's break the term into two parts: AI and Agents.
What Are Agents?
Agents, in general, are information assistants.
- Human agents include call center representatives who assist with information requests or perform tasks like booking flights and hotels.
- Machine agents: Chatbots that follow pre-programmed scripts can be considered agents, but they are not AI Agents.
For a system to be considered an AI Agent, it must incorporate AI capabilities.
- Voice bots with AI-powered speech recognition but fixed response scripts don't fully qualify as AI Agents.
- Virtual assistants like Siri, Alexa, and Google Assistant represent early versions of AI Agents. They can recognize speech, analyze queries, personalize responses, and provide relevant information.
The Rise of AI Agents with Generative AI
With the advent of Generative AI (also known as Foundation AI), AI Agents have evolved significantly. However, before diving deeper into AI Agents, it's essential to understand Machine Learning and AI Engineering.
The Role of Machine Learning in AI
Before ChatGPT, discussions about AI often focused on Machine Learning. Building AI requires extensive training (or "training datasets").
For example, thousands of labeled images had to be manually annotated to develop an AI that recognizes an image of a fried egg. This process required significant investment in:
- Computing power
- Machine learning engineers
- Large labeled datasets
- Time and financial resources for adjustments and upgrades
AI Becomes More Accessible
With the rise of ChatGPT and other generative AI models, integrating AI into applications has become much more accessible:
- There is no need for extensive data training as pre-trained models are available.
- AI models are trained on massive datasets, offering better accuracy and flexibility.
- AI as a Service (AIaaS) has become mainstream, enabling developers to integrate AI into applications with basic programming knowledge quickly.
AI Agents: The Core, the Optimization, and the Interface
To better understand how AI Agents function, consider them as having three main components:
- The Core (Foundation AI): The underlying AI model (e.g., ChatGPT, Gemini) trained on vast amounts of data.
- The Optimization Layer: Techniques such as Prompt Engineering, RAG (Retrieval-Augmented Generation), and Fine-tuning help tailor AI responses for specific needs.
- The Interface (User Interaction Layer): The visible part of AI Agents, including chatbots and voicebots, which interact with users through text, voice, images, music, or videos.
Challenges and Considerations for AI Agents
Despite their advancements, AI Agents still face challenges:
- Hallucination (Misinformation): AI-generated responses can sometimes be incorrect but presented as factual.
- Lack of Real-Time Data: AI models typically rely on previously trained datasets and may not have up-to-date information.
- Limited Domain-Specific Knowledge: AI models may not have detailed or proprietary information unless specifically trained.
AI engineers implement prompt engineering, RAG, and fine-tuning to address these issues and make AI agents more innovative and relevant.
What's Next?
In the following article of this series, we'll explore Prompt Engineering, RAG, and Fine-Tuning in detail. These techniques are crucial in refining AI Agents and making them more effective for specific business applications.
Stay tuned!